|
Home
News
People
Research
Publications
Courses
Seminars
Data & Code
Contact Us
Calendar
Other Links
|
|
|
|
Efficient Inference Algorithm for vision tracking
Members: Minwoo Park, Yanxi Liu and Robert T. Collins
Project Descriptions
A mechanism for efficient mean-shift belief propagation (MSBP) is introduced. The novelty of our work is to use mean-shift as a mode-seeking mechanism operating on samples and weights generated within the belief propagation framework. While the recently proposed nonparametric belief propagation (NBP) is well-suited for continuous hidden variable1 problems, computation is slow due to the particle filter sampling process. Although the continuous variable space can be discretized, this leads to inefficient computation via discrete belief propagation. Our new method uses mean-shift mode-seeking within the discrete grid of weights and samples, and needs a significantly smaller number of samples than particle filtering, reducing computational cost as compared to NBP.
Formal verification is difficult, but we also observe that our approach converges when discrete belief propagation with continuous compatibility functions does not.
Extensive experiments on challenging multi-target tracking applications demonstrate smooth and efficient tracking results using the proposed algorithm.
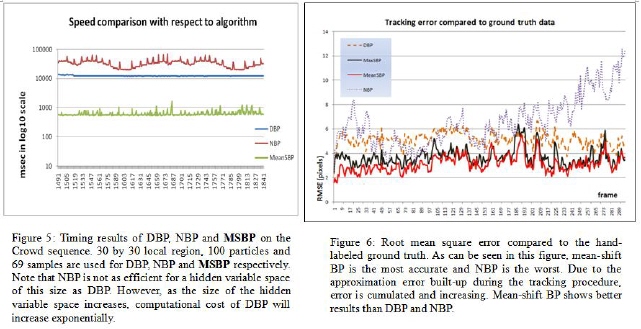
|
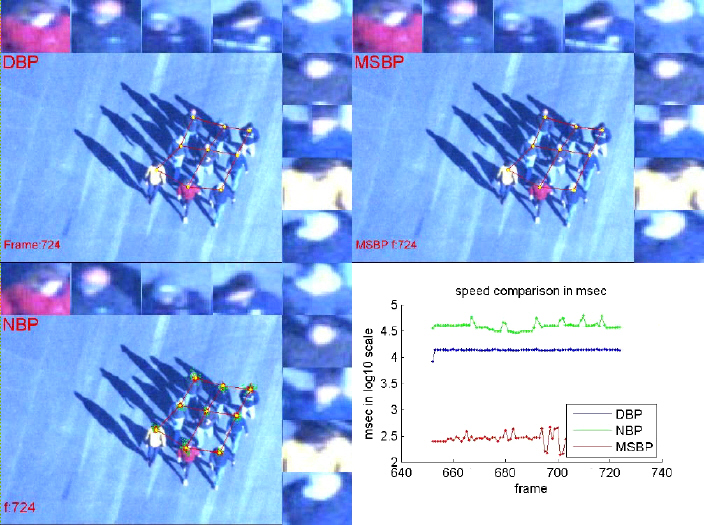 |
Figure 6. Tracking Comparison in terms of speed, accuracy and stability
|
|
Figure 7. Tracking Comparison for articulated body tracking
|
|
|