Members:
Description:
This work is a reaction to the poor performance of symmetry detection algorithms on real-world images, benchmarked since CVPR 2011. Our systematic study reveals significant difference between human labeled (reflection and rotation) symmetries on photos and the output of computer vision algorithms on the same photo set. We exploit this human-machine symmetry perception gap by proposing a novel symmetry-based Turing test. By leveraging a comprehensive user interface, we collected more than 78,000 symmetry labels from 400 Amazon Mechanical Turk raters on 1,200 photos from the Microsoft COCO dataset. Using a set of ground-truth symmetries automatically generated from noisy human labels, the effectiveness of our work is evidenced by a separate test where over 96% success rate is achieved. We demonstrate statistically significant outcomes
for using symmetry perception as a powerful, alternative, image-based reCAPTCHA.
Samples:
Original Image |
Human Labels |
Ground Truth Labels |
|
|
|
|
|
|
Figure 1. Sample images showing labels and ground truth extraction. |
Published Papers
Symmetry reCAPTCHA
Christopher Funk and Yanxi Liu
Proceedings of the IEEE Conference on Computer Vision and Pattern Recognition. p. 5165-5174.
2016.
PDF Link
Published Abstract:
LabelMe Symmetry: a tool for human symmetry perception.
Christopher Funk and Yanxi Liu
Vision Sciences Society,
St. Pete, Florida. 2016
Even at a young age, most people can identify where symmetry resides within an image. However, there are no open datasets on human symmetry labels on real world images. There have been a few datasets created to compare symmetry detection algorithms but they have been limited and only labeled by a few individuals, preventing in depth study of symmetry perception on real images. We utilize an in-house crowdsourcing tool and the publicly available Microsoft COCO dataset to collect symmetry labels on real world images deployed on Amazon Mechanical Turk (AMT). The tool strives not to influence people's understanding of symmetry. For relatively low cost, the crowdsourcing tool has been able to create a dataset of 78,724 symmetry labels on over 1,000 images by 300+ AMTs from all over the world. To our knowledge, this is the largest dataset of symmetry labels for both perceived rotation symmetries and reflection (mirror) symmetries on natural photos. The labeled symmetries are then clustered automatically to find the statistical consensus on each image. Based on these consensuses, the Precision-Recall (PR) curves are analyzed between the different gender, education level and age group of the AMTs. No statistical difference is found between male and female AMTs, while statistically significant differences in symmetry perception are found between different education levels and age-groups. For example, we demonstrate p-value < 0.001 for AMTs of age 51-71 with all other age groups in PR performance, and doctoral/professional AMTs with all other education levels. The younger AMTs (less than or equal to 20 years old) and AMTs with high school education level, respectively, perform the best among all categories.
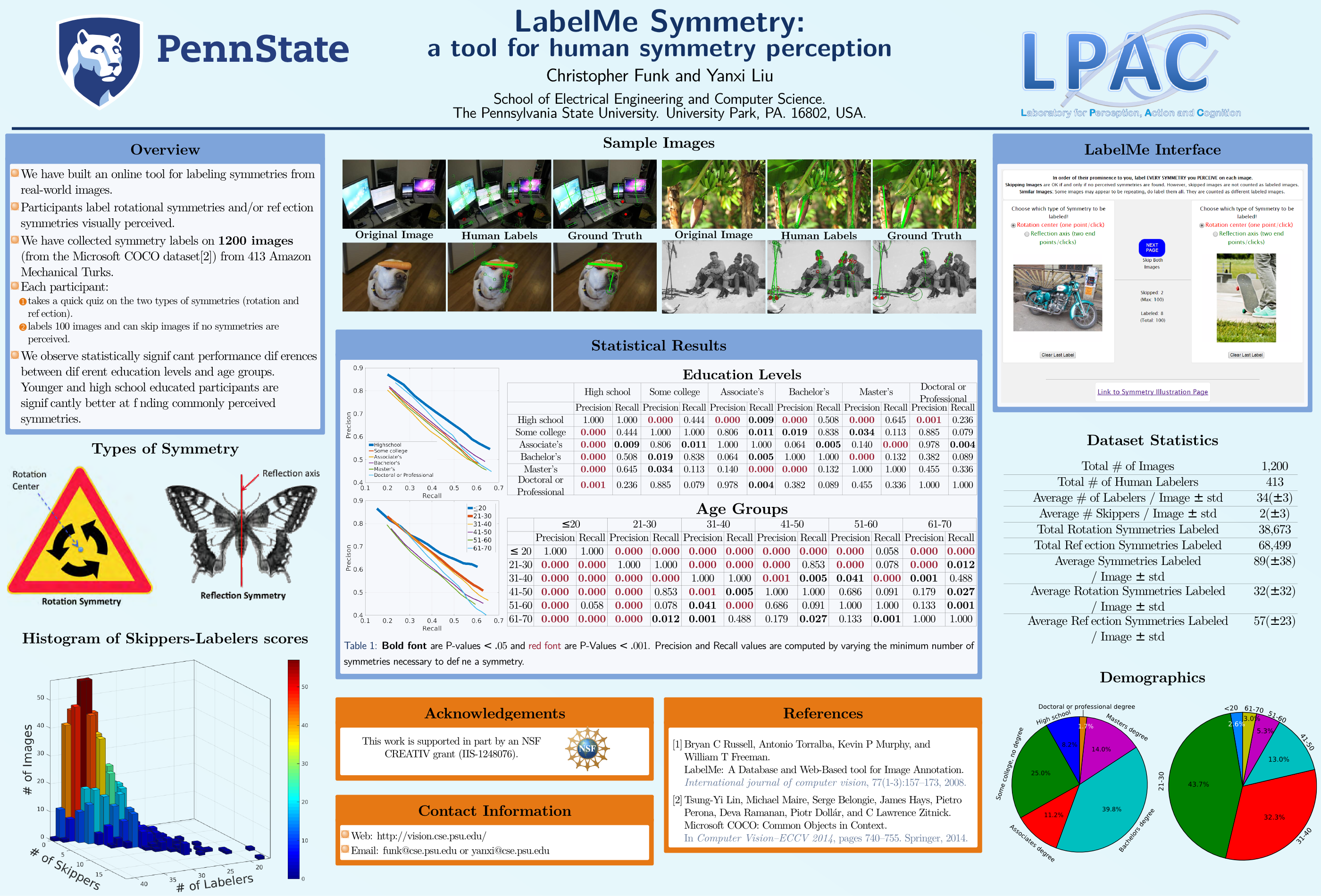 |
Poster [PDF - 4.1MB] |
Acknowledgment:
This project is a Penn State and Stanford University collaboration funded by a National Science Foundation (NSF) Creative Research Award for Transformative Interdisciplinary Ventures (CREATIV)
INSPIRE: Symmetry Group-based Regularity Perception in Human and Computer Vision (NSF IIS-1248076)