Members: Gang Zheng, Yanxi Liu and Robert T. Collins
Collaborators: Dan T.D. Nguyen
Past Members: Chenping Yu, Somesh Kashyap and Diana Koshy
![]() |
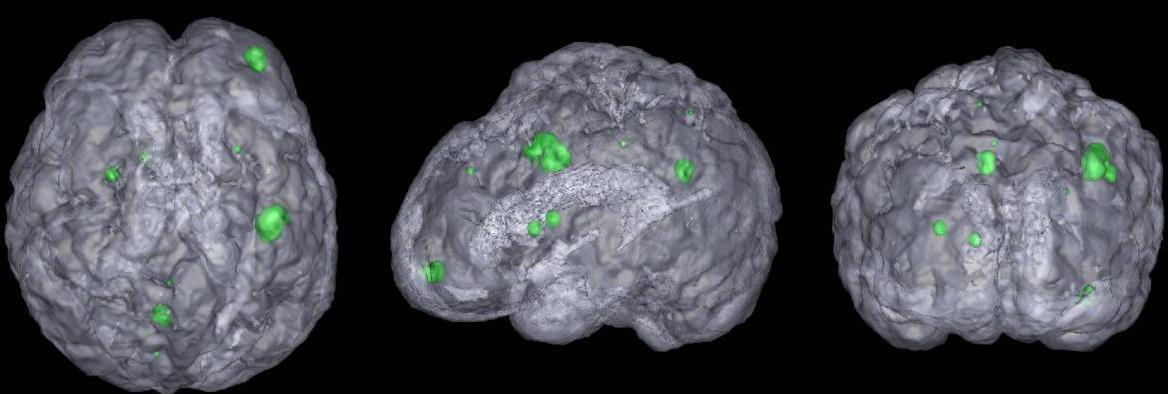
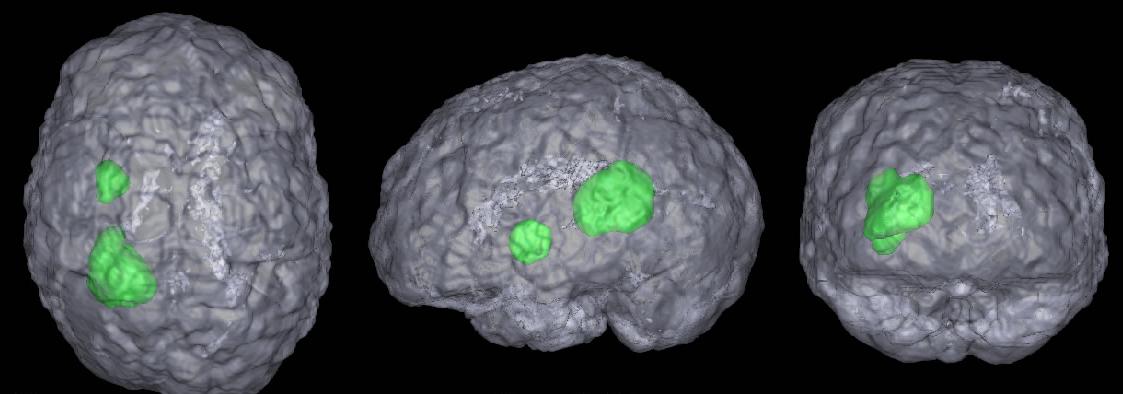
3D Blob based Brain Tumor Detection and Segmentation in MR Images
Citation
![]() | Chen-Ping Yu, Guilherme Ruppert, Robert Collins, Dan Nguyen, Alexandre Xavier, and Yanxi Liu. 3D Blob based Brain Tumor Detection and Segmentation in MR Images, IEEE Internation Symposium on Biomedical Imaging (ISBI) Beijing, China, 2014. [PDF] |
Abstract
Automatic detection and segmentation of brain tumors in 3D MR neuroimages can significantly aid early diagnosis, surgical planning, and follow-up assessment. However, due to diverse location and varying size, primary and metastatic tumors present substantial challenges for detection. We present a fully automatic, unsupervised algorithm that can detect single and multiple tumors from 3 to 28,079 mm3 in volume. Using 20 clinical 3D MR scans containing from 1 to 15 tumors per scan, the proposed approach achieves between 87.84% and 95.30% detection rate and an average end-to-end running time of under 3 minutes. In addition, 5 normal clinical 3D MR scans are evaluated quantitatively to demonstrate that the approach has the potential to discriminate between abnormal and normal brains.
Statistical Asymmetry-based Brain Tumor Segmentation from 3D MR Images
Citation
![]() | Chenping Yu, Guilherme C. S. Ruppert, Dan T. D. Nguyen, Alexandre X. Falcao, and Yanxi Liu. Statistical Asymmetry-based Brain Tumor Segmentation from 3D MR Images, 5th international joint conference on biomedical engineering systems and technologies, 2012. [PDF] |
Abstract
The precise segmentation of brain tumors from MR images is necessary for surgical planning. However, it is a tedious task for the medical professionals to process manually. The performance of supervised machine learning techniques for automatic tumor segmentation is time consuming and very dependent on the type of the training samples. Brain tumors are statistically asymmetrical blobs with respect to the mid-sagittal plane (MSP) in the brain and we present asymmetry-based, novel, fast, fully-automatic and unsupervised framework for 3D brain tumor segmentation from MR images. Our approach detects asymmetrical intensity deviation of brain tissues in 4 stages: (1) automatic MSP extraction, (2) asymmetrical slice extraction for an estimated tumor location, (3) region of interest localization, and (4) 3D tumor volume delineation using a wa- tershed method. The method has been validated on 17 clinical MR volumes with a 71.23%±27.68% mean Jaccard Coeffcient.
Supervised Machine Learning for Brain Tumor Detection in Structural MRI
Citation
![]() |
|
Abstract
Brain tumor detection from MRI data is tedious for physicians and challenging for computers. Detection of small tumors, which often form early primary neoplastic or metastatic disease, is particularly difficult and has only recently been a focus of state-of-the-art work. Starting with skull-stripping and midsagittal plane alignment, tumor candidates as blobby structures are obtained by 3D image convolution using multi-scale LoG filters. We then compute the blob shape, compactness, asymmetry and intensity statistics, generating a set of 21 features (9.5 min). For training, Sequential Forward Selection, wrapped around a Quadratic Discriminant Analysis classifier, is performed on the feature set (8 sec). For testing, 5 classifiers (LDA, QDA, Naive Bayes (linear, quadratic), Mahalanobis) are used in the selected feature space (average 8.2 features, 1.3 sec). Our data consists of 20 T1- weighted enhanced brain MRIs with a total of 86 tumors (2-38 mm diameter). We perform 100 random training- testing splits where 85% of the tumors and an equal number of non-tumors are used for training; the remaining data is used for testing. The average sensitivity and specificity rates are 97.5%(+-4.7) and 94.7%(+-1.4) respectively.
links to earlier work
http://www.cs.cmu.edu/~yanxi/images/medical_image.html |
http://www.ri.cmu.edu/research_project_detail.html?project_id=281&menu_id=261 |